The first rule of data:
And, your reaction might be: Of course!- Don't ask for data if you don't know what you are going to do with it
- Don't ask for data which you can not use or act upon
But, alas, in the PMO there are too many incidents of reports, data accumulation, measurements, etc which are PMO doctrine, but in reality, there actually is no plan for what to do with it. Sometimes, it's just curiosity; sometimes it's just blind compliance with a data regulation; sometimes it's just to have a justification for an analyst job.
The test:
If someone says they need data, the first questions are:
- What are you going to do with the data?
- How does the data add value to what is to be done
- Is the data quality consistent with the intended use or application (**), and
- Is there a plan to effectuate that value-add (in other words, can you put the data into action)?
And how much data?
Does the data inquisitor have a notion of data limits: What is enough, but not too much, to be statistically significant (*), informative for management decision making, and sufficient to establish control limits?
And information?
Well, the usual definition is that information is data, perhaps multiple data, integrated with context, and interpreted for the current situation.
So, the rule can be extended: If there are not means to process data into information, is the data necessary to be collected?
Bottom line: To state the obvious: always test a request for data collection for value-add before spending resources!
_____________
(*) Statistically significant: Enough data to distinguish between a 'random or chance outcome', and an outcome that is really from an event, factor, or stimulus of interest. As a practical matter for the uses of PM, ten independent data points is usually enough to establish statistical significance. Other disciplines may require more.
(**) Data quality: To add value, the data must be timely vis a vis the context, as complete as practical (nothing left out), consistent over time and space, more unique than redundant, sufficiently accurate in a metric sense, and void -- to the extent possible -- of qualitative biases. The latter is a tall order since all human reasoning is biased in some way or another.
Like this blog? You'll like my books also! Buy them at any online book retailer!
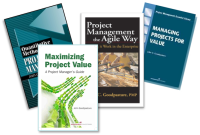