Is keeping project history valuable? Doesn't every project office have at least cost history? Isn't all parametric pricing based on history?
- Yes, Maybe, Yes .... respectively, to the above!
- And, could there be parametric estimating without history (*)? No, definitely not.
- Or, could there be project, event, or risk statistics without history? (**) No!
Ooops, perhaps everyone does not agree:
"History can not be explained deterministically and it can not be predicted because it is chaotic.
So many forces are at work and their interactions are so complex that extremely small variations in the strength of forces and the way they interact predict huge differences in outcomes....
Not only that, but history is what is called a level two chaotic system (***). ... Level two chaos is chaos that reacts to predictions about it, and therefore can never be predicted accurately"
Yuval Harari
And, so the take away on this is what?
That history is useless for predicting an outcome? Or, that one historical outcome could easily have been another, quite different, except for some favorable interactions -- thus, who knows what might happen the next time around?
Or, even more intriguing is the last point: a prediction actually changes the predicted outcome. Somewhat like the oft encountered conundrum that a measurement or observation may change that which is measured or observed. (****)
And, of course, there is the timeless nemesis: causation vs correlation vs coincidence.
----------------
Or, even more intriguing is the last point: a prediction actually changes the predicted outcome. Somewhat like the oft encountered conundrum that a measurement or observation may change that which is measured or observed. (****)
And, of course, there is the timeless nemesis: causation vs correlation vs coincidence.
- Causation: A causes C
- Correlation: A causes some reaction in B which causes some reaction in C (correlation has a third party in most cases, though B may be hidden and hard to discern)
- Coincidence: Stuff happens
----------------
(*) Parametric estimating: $X per page; $X per line of code; $X per linear foot, etc
(**) A statistic is a calculation made from observed or measured values, like the average of all the salaries on a team. Statistics are 'backward' looking in the sense that all the data in the calculation comes from history.
(***) There are two main classifications of chaos, explains Daniel Miessler:
First Order Chaos doesn’t respond to prediction. The example [ ] is the weather. If you predict the weather to some level of accuracy that prediction will hold because the weather doesn’t adjust based on the prediction itself.
Second Order Chaos is infinitely less predictable because it does respond to prediction. Examples include things like stocks and politics.
(****) As an example, when probing sensitive electronic circuits, the probe itself can change the performance of the circuit.
Like this blog? You'll like my books also! Buy them at any online book retailer!
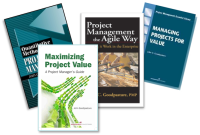